Within the three-dimensional space of cells and tissues, intricate biological processes operate with continuous precision and order, sustaining the fundamental functions essential for life. Light-sheet microscopy (LSM), currently the most suitable modality for three-dimensional imaging of multicellular and large-volume specimens, employs two orthogonally arranged objective lenses to perform sheet illumination and wide-field detection separately. This configuration enhances the optical sectioning capability while significantly reducing photobleaching and phototoxicity in live samples during 3D imaging. Nevertheless, constrained by its specialized objective lens configuration, LSM typically exhibits lower spatial resolution compared to conventional methods such as confocal microscopy. Enhancing its resolution remains a longstanding challenge in the field.
To address this challenge, Nobel Laureate in Chemistry Eric Betzig pioneered the lattice light-sheet microscope (LLSM) in 2014. By integrating this innovation with structured illumination microscopy (SIM), he developed the lattice light-sheet structured illumination microscopy (LLS-SIM). The LLS-SIM system achieves approximately 1.5-fold resolution enhancement along the structured illumination direction, representing the current state-of-the-art in microscopic imaging technology. In contrast to conventional SIM, LLS-SIM generates structured illumination in only one direction, resulting in anisotropic spatial resolution. This unidirectional approach induces artifacts in non-super-resolved dimensions and consequently constrains precise 3D subcellular dynamic detection.
In recent years, intelligent computational methods, particularly deep learning, have exerted revolutionary impacts on the development of optical microscopy. Through the co-optimization of optical imaging systems and intelligent algorithms, we can substantially overcome the inherent spatiotemporal bandwidth limitations in optical system design, enabling ultrahigh-speed, super-resolution, and long-term live-cell fluorescence microscopy. A critical frontier challenge in 3D super-resolution microscopy lies in overcoming the performance limitations of current imaging tools through an interdisciplinary combination of optical systems and artificial intelligence. This breakthrough would enable dynamic biological processes to be observed with true 3D visualization and isotropic subcellular resolution.
A collaborative study between Professor Dong Li's research group from the School of Life Sciences at Tsinghua University and Academician Qionghai Dai's team from the Department of Automation, titled "Fast-adaptive super-resolution lattice light-sheet microscopy for rapid, long-term, near-isotropic subcellular imaging", was published in Nature Methods on April 29, 2025. By integrating multiple technological innovations - including virtual structured illumination, mirror-enhanced dual-view detection, and Bayesian dual-view fusion reconstruction - this approach extends the conventional LLS-SIM's one-dimensional super-resolution capability to full X-Y-Z dimensions. The system achieves near-isotropic imaging resolution of 120nm laterally and 160nm axially, without compromising imaging speed or photon efficiency. Furthermore, by deeply integrating meta-learning strategies with the system's data acquisition process, the adaptive deployment from training data collection to deep learning model optimization can be completed in just three minutes. This seamless integration achieves near 'zero-barrier' implementation of AI tools in practical biological experiments.
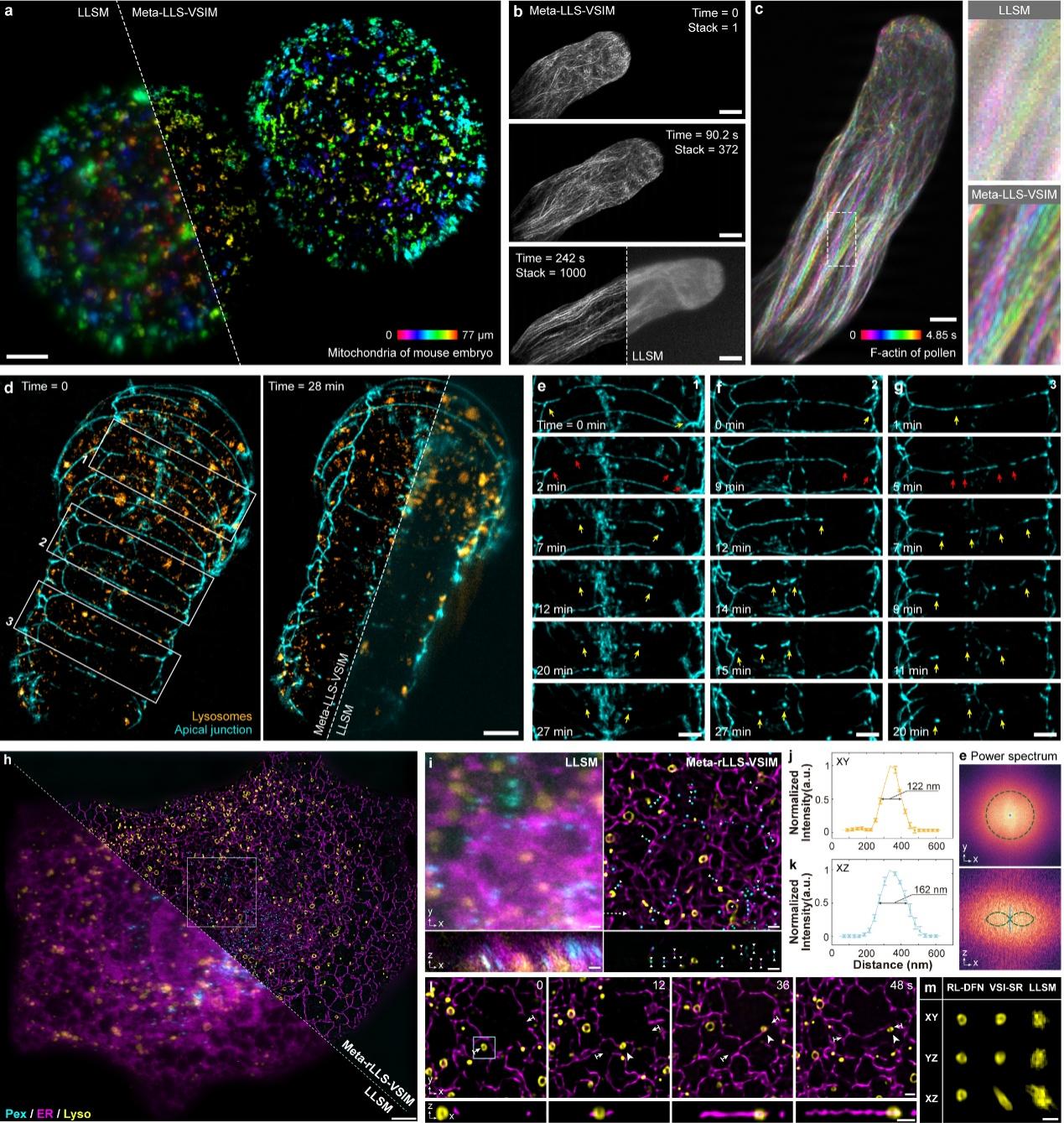
Fig1 Demonstration of rapid five-dimensional super-resolution live-cell imaging using Meta-rLLS-VSIM
To demonstrate Meta-rLLS-VSIM's capability for rapid five-dimensional (wavelength-X-Y-Z-time) super-resolution live-cell imaging, the collaborative team performed long-term super-resolution observations on thick, large-volume specimens, including mouse embryos (Fig. a), plant pollen tubes (Figs. b, c), and C. elegans embryos (Figs. d-g). Combining the physical optical sectioning capability of lattice light-sheet illumination with near-isotropic 3D super-resolution imaging, Meta-rLLS-VSIM clearly revealed key biological processes, including tip-polarized growth in pollen tubes and plasma membrane fusion during C. elegans embryonic development.
Furthermore, the collaborative team employed Meta-rLLS-VSIM to perform rapid (acquiring full-cell three-channel data every 8 seconds), long-term (>800 time points), near-isotropic super-resolution imaging of intact COS-7 cells (Figs. h-m). This enabled precise quantitative analysis of 3D spatial distribution patterns of various organelles and their spatiotemporal coordination mechanisms with the cytoskeleton. Leveraging the system's high spatiotemporal resolution and prolonged observation window, the collaborative team captured novel phenomena including "hitchhiking" between microtubules and lysosomes, as well as mitochondrial fission driven by mechanical forces from lysosomal movement. These findings demonstrate Meta-rLLS-VSIM's potential for uncovering new biological phenomena and mechanisms.
Collectively, Meta-rLLS-VSIM achieves hardware-software co-optimization through two key innovations: (1) hardware upgrades featuring a reflection-enhanced dual-view lattice light-sheet microscope and a meta-learning-enabled rapid adaptive deployment framework, and (2) algorithmic breakthroughs incorporating virtual structured illumination and a reinforcement learning (RL)-based dual-cycle fusion network. This synergistic approach significantly enhances overall imaging performance. This technological breakthrough establishes a novel methodological framework for advancing fundamental disciplines such as cell biology and neuroscience. Moving forward, it holds significant potential to empower life science researchers in discovering, understanding, and exploring diverse biological phenomena from a more comprehensive, multidimensional perspective.
Professor Dong Li from Tsinghua University's School of Life Sciences and Professor Qionghai Dai from the Department of Automation served as corresponding authors of this study. The co-first authors include Dr. Chang Qiao (Postdoctoral Fellow, Tsinghua University), Associate Researcher Ziwei Li (Fudan University), Dr. Zongfa Wang (Postdoctoral Fellow, Institute of Biophysics, Chinese Academy of Sciences), Yuhuan Lin (Ph.D. candidate, Institute of Biophysics, Chinese Academy of Sciences), and Dr. Chong Liu (Postdoctoral Fellow, École Polytechnique Fédérale de Lausanne)