The SARS-CoV-2 virus is the causal pathogen for the worldwide COVID-19 pandemic (Perlman, 2020). To date, SARS-CoV-2 has infected more than 100 million people with more than 2.5 million deaths, causing tremendous damage to the global human society. SARS-CoV-2 is a single-stranded RNA virus, and its viral RNA is a key component in regulating host infection: the tiny SARS-CoV-2 genome (and the limited proteome it encodes) relies heavily on interactions with proteins in host cells—the so-called “host factors”—to complete the viral lifecycle (Gordon et al., 2020; Schmidt et al., 2020). Thus, understanding the molecular structure of SARS-CoV-2 RNA and identifying the host factors that interact with it can support the development of efficacious drugs to treat COVID-19.
Recently, Prof. Qiangfeng Cliff Zhang's group at Tsinghua University School of Life Sciences, in collaboration with Prof. Jianwei Wang's group at the Chinese Academy of Medical Sciences and Prof. Qiang Ding's group at Tsinghua University School of Medicine, resolved the SARS-CoV-2 RNA genome structure in infected human cells. This in vivo structural data informed a deep learning Artificial Intelligence tool, also developed by the Zhang group, to predict binding of host factor proteins on SARS-CoV-2 RNA. Strikingly, the research team later experimentally confirmed that several of these predicted host proteins are vulnerable to chemical inhibition of some already approved FDA-approved drugs. That is, these re-purposed drugs disrupt the capacity of the SARS-CoV-2 infections in cultured cells. These scientific, technological, and medical advances together shed new light on coronaviruses and have revealed multiple potential candidate therapeutics for COVID-19 treatment (Figure 1).
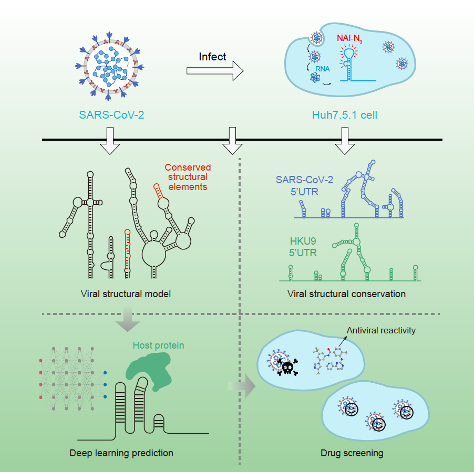
Figure 1 | Resolving SARS-CoV-2 RNA structure, identifying conserved RNA structures, discovering interactions with host proteins, and screening for antiviral drug candidates (adapted from Sun et. al., 2021a).
RNA structure is the basis of RNA function and regulation. Previous studies have resolved a large number of RNA structures by X-ray crystallography, nuclear magnetic resonance, and cryo-electron microscopy, revealing mechanistic details about RNA function. In recent years, innovative techniques that combine in cellulo RNA chemical modifications with high-throughput sequencing technologies have supported the characterization of RNA secondary structures in vivo at a transcriptome-wide scale (Spitale et al., 2015). These advances for RNA systems biology have started to reveal how RNA structure governs post-transcriptional biological regulation.
Qiangfeng Cliff Zhang's research program has been squarely focused on RNA structure since its inception, initially focusing on new technology development and applications and more recently using these tools to powerfully illustrate how understanding RNA structure can drive scientific and medical insights (Sun et al., 2019). In a previous work, The Zhang group resolved the RNA structural landscape of the ZIKA virus (”ZIKV”, including epidemic Asian strains and non-epidemic African strains) in infected cells. Notably, they discovered a long-range intramolecular interaction specific for the epidemic Asian ZIKV strains that contributes to the enhanced infectivity of these strains. The study highlighted the complexity and functional relevance of RNA virus structure during infection, elucidated a novel molecular mechanism based on RNA secondary structure, and provided an empirical structural foundation to support the development of effective therapeutics (Li et al., 2018).
The Zhang group is also active in innovating new AI-based methods to tackle complex biological problems. In a parallel research project, the group developed an Artificial Intelligence tool, PrismNet, which uses deep neural networks to predict the binding sites of RNA binding proteins (RBPs) based on integration of in vivo RNA structural information with RBP binding information for corresponding cell lines (Figure 2). A particularly salient result from this is the demonstration that intracellular RNA structure information profoundly improves prediction accuracy, especially for the prediction of binding sites that undergo dynamic changes in specific cellular contexts. Cumulative efforts over years of research based on the CLIP technique have now generated approximately 200 RBP transcriptome binding profiles, and these collectively represent a foundational data resource for studying RNA regulation and RBP functions. It is clear that PrismNet can be harnessed to greatly expand the scope and biological discovery utility of these data resources. As a specific example: for any RBP with CLIP experimental data from a certain biological context, we now know that PrismNet can informatively extrapolate binding information to any other cellular condition(s) for which RNA structure data has been acquired (Sun et al., 2021b).
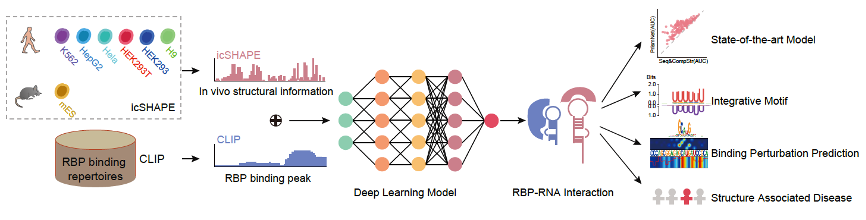
Figure 2 | Construction and application of the deep learning tool PrismNet (adapted from Sun et. al., 2021b)
Upon the outbreak of COVID-19, the Zhang group acted quickly and undertook collaborations with Prof. Jianwei Wang's group at the Peking Union Medical College and with Prof. Qiang Ding's group at Tsinghua. These collaborations have been focused both on deepening our understanding of the molecular virology of SARS-CoV-2 infection and on discovering effective antiviral drugs. Building upon their innovative technologies, the team used icSHAPE (previously co-invented by Dr. Zhang) to resolve the genome-wide secondary structure of RNA of SARS-CoV-2 in infected cells.
Through structural analysis, the team found an abundance of conserved RNA structural elements and also verified the functional impacts of related RNA structural elements on the viral lifecycle, using experimental techniques like antisense oligo (ASO) blocking of RNA structure, as well as viral structural mutation to disrupt and complementary mutations to restore structures. They subsequently verified that several of the newly discovered conserved RNA structural elements do contribute to viral infection. More excitingly, the team used the aforementioned PrismNet AI tool and successfully predicted multiple host proteins that interact with SARS-CoV-2 RNA. They experimentally validated the physical and functional interactions of several proteins predicted to bind viral RNA. Demonstrating the translational relevance and application of these very “basic” research findings, they found that several of the identified host proteins are vulnerable to disruption by drugs. That is, repurposed FDA-approved drugs can be used to chemically inhibit host proteins to significantly reduce SARS-CoV-2 infection in cultured cells, thereby revealing multiple potential candidate therapeutics for treating COVID-19 (Sun et al., 2021a).
The study which characterized the SARS-CoV-2 RNA genome structure and demonstrated drug repurposing was published in Cell on Feb 9, in a paper titled "In vivo structural characterization of the SARS-CoV-2 RNA genome identifies host proteins vulnerable to repurposed drugs” (https://www.cell.com/cell/fulltext/S0092-8674(21)00158-6). The study which developed the AI tool for the prediction of in vivo RNA-protein interactions was published in Cell Research on Feb 23, in a paper titled " Predicting dynamic cellular protein-RNA interactions using deep learning and in vivo RNA structure" (https://doi.org/10.1038/s41422-021-00476-y).
These efforts were led and coordinated by Prof. Qiangfeng Cliff Zhang from Tsinghua University School of Life Sciences, in collaboration with Prof. Jianwei Wang from the Chinese Academy of Medical Sciences and Prof. Qiang Ding from Tsinghua University School of Medicine. Core members of the team also included Drs. Lei Sun (from Tsinghua) as well as Jian Rao and Lili Ren (both from PUMC); and PhD students Pan Li, Kui Xu, Xiaohui Ju, and Wenze Huang (all from Tsinghua). These research efforts were funded by the National Natural Science Foundation of China, the Key Research and Development Program of the Ministry of Science and Technology, Tsinghua University Breeze Fund, the Beijing Advanced Innovation Center for Structural Biology, and the Tsinghua-Peking Center for Life Science.
References:
Gordon, D.E., Jang, G.M., Bouhaddou, M., Xu, J., Obernier, K., White, K.M., O'Meara, M.J., Rezelj, V.V., Guo, J.Z., Swaney, D.L., et al. (2020). A SARS-CoV-2 protein interaction map reveals targets for drug repurposing. Nature 583, 459-468.
Li, P., Wei, Y., Mei, M., Tang, L., Sun, L., Huang, W., Zhou, J., Zou, C., Zhang, S., Qin, C.F., et al. (2018). Integrative Analysis of Zika Virus Genome RNA Structure Reveals Critical Determinants of Viral Infectivity. Cell Host Microbe 24, 875-886 e875.
Perlman, S. (2020). Another Decade, Another Coronavirus. N Engl J Med 382, 760-762.
Schmidt, N., Lareau, C.A., Keshishian, H., Ganskih, S., Schneider, C., Hennig, T., Melanson, R., Werner, S., Wei, Y., Zimmer, M., et al. (2020). The SARS-CoV-2 RNA-protein interactome in infected human cells. Nat Microbiol.
Spitale, R.C., Flynn, R.A., Zhang, Q.C., Crisalli, P., Lee, B., Jung, J.W., Kuchelmeister, H.Y., Batista, P.J., Torre, E.A., Kool, E.T., et al. (2015). Structural imprints in vivo decode RNA regulatory mechanisms. Nature 519, 486-490.
Sun, L., Fazal, F.M., Li, P., Broughton, J.P., Lee, B., Tang, L., Huang, W., Kool, E.T., Chang, H.Y., and Zhang, Q.C. (2019). RNA structure maps across mammalian cellular compartments. Nature structural & molecular biology 26, 322-330.
Sun, L., Li, P., Ju, X., Rao, J., Huang, W., Zhang, S., Xiong, T., Xu, K., Zhou, X., Ren, L., et al. (2021a). In vivo structural characterization of the whole SARS-CoV-2 RNA genome identifies host cell target proteins vulnerable to re-purposed drugs. Cell https://doi.org/10.1016/j.cell.2021.02.008.
Sun, L., Xu, K., Huang, W., Yang, Y.T., Li, P., Tang, L., Xiong, T., and Zhang, Q.C. (2021b). Predicting dynamic cellular protein-RNA interactions using deep learning and in vivo RNA structure. Cell Research, https://doi.org/10.1038/s41422-41021-00476-y.